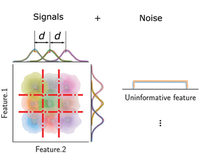
Quantifying the impact of uninformative features on the performance of supervised classification and dimensionality reduction algorithms
Abstract
Machine learning approaches have become critical tools in data mining and knowledge discovery, especially when attempting to uncover relationships in high-dimensional data. However, researchers have noticed that a large fraction of features in high-dimensional datasets are commonly uninformative (too noisy or irrelevant). Because optimal feature selection is an NP-hard task, it is essential to understand how uninformative features impact the performance of machine learning algorithms. Here, we conduct systematic experiments on algorithms from a wide range of taxonomy families using synthetic datasets with different numbers of uninformative features and different numbers of patterns to be learned. Upon visual inspection, we classify these algorithms into four groups with varying robustness against uninformative features. For the algorithms in three of the groups, we find that when the number of uninformative features exceeds the number of data instances per pattern to be learned, the algorithms fail to learn the patterns. Finally, we investigate whether increasing the distinguishability of patterns or adding training instances can mitigate the effect of uninformative features. Surprisingly, we find that uninformative features still cause algorithms to suffer big losses in performance, even when patterns should be easily distinguishable. Analyses of real-world data show that our conclusions hold beyond the synthetic datasets we study systematically.